The digitization of society brings about a structural change of the public sphere and the private, in which algorithms – quite literally – play a decisive role. We all interact in and with socio-technical systems, e.g. social media, search engines, online stores, job application platforms, news and information platforms. In these systems, algorithms play a major role in deciding which content, groups, people or institutions are presented or recommended to us and how they are being priorized. Algorithms often take the concrete behavior of users as a starting point and thus create a complex recursive interaction between the operating algorithm and human action or experience. With this, artificial intelligence, algorithms and automated processes create dynamics that might not be perceivable by users but do create social structures that substantially influence our individual lives and society as a whole. Whether or not these consequences are desirable can only be discussed and evaluated if we know precisely how digital technologies, the Web and the algorithms therein shape social structures.
This is why GESIS studies the mechanisms of socio-technical systems in order to understand the social change they bring about and to improve the basis for informed and "good" decisions. We do this through collecting digital behavioral data on societal issues, conducting online experiments to analyze behavioral patterns and their susceptibility in digital environments, and developing analytical tools. One of the most pressing social issues is inequality. Algorithms can reinforce existing social inequality or generate new distortions or discrimination. We investigate how distortions (e.g. gender bias) occur in digital practice and how, on the other hand, algorithms and AI can be used to counteract structural inequality and injustice or misinformation.
- Wieland, Mareike, Gerret von Nordheim, and Katharina Kleinen-von Königslöw. 2021. "One recommender fits all? An exploration of user satisfaction with text-based news recommender systems. ." Media and Communication 9 (4): 208-221. doi: https://doi.org/10.17645/mac.v9i4.4241.
- Strohmaier, Markus, Lukas Moldon, and Johannes Wachs. 2021. "How Gamification Affects Software Developers: Cautionary Evidence from a Natural Experiment on GitHub." In 43rd IEEE/ACM International Conference on Software Engineering, ICSE 2021, Madrid, Spain, 22-30 May 2021, 549-561. IEEE Computer Society. doi: https://doi.org/10.1109/ICSE43902.2021.00058.
- Neuhäuser, Leonie, Felix Stamm, Florian Lemmerich, Michael Schaub, and Markus Strohmaier. 2021. "Simulating systematic bias in attributed social networks and its effect on rankings of minority nodes." Applied Network Science 6 86. doi: https://doi.org/10.1007/s41109-021-00425-z.
- Sen, Indira, Fabian Flöck, Katrin Weller, Bernd Weiß, and Claudia Wagner. 2022. "Applying a total error framework for digital traces to social media research." In Handbook of Computational Social Science. Volume 2: Data science, statistical modelling, and machine learning methods, edited by Uwe Engel, Anabel Quan-Haase, Sunny Xun Liu, and Lars Lyberg, 127-139. Routledge.
- Soldner, Felix, Leonie Maria Tanczer, Daniel Hammocks, Isabel Lopez-Neira, and Shane Johnson. 2021. "Using machine learning methods to study technology-facilitated abuse: Evidence from the analysis of UK crimestoppers’ text data." In The Palgrave handbook of gendered violence and technology, edited by Anastasia Powell, Asher Flynn, and Lisa Sugiura, 481-503. Basingstoke u.a.: Palgrave Macmillan. doi: https://doi.org/10.1007/978-3-030-83734-1_24.
Title | Start | End | Funder |
---|---|---|---|
Political polarization and individualized online information environments: A longitudinal tracking study
(POLTRACK)
|
2022-01-01 | 2025-08-30 | SAW (Leibniz) |
NFDI for Data Science and Artificial Intelligence
(NFDI4DS)
|
2021-10-01 | 2026-09-30 | DFG |
Dehumanization Online: Measurement and Consequences (Professorinnenprogramm)
(DeHum)
|
2021-01-01 | 2026-09-30 | SAW (Leibniz) |
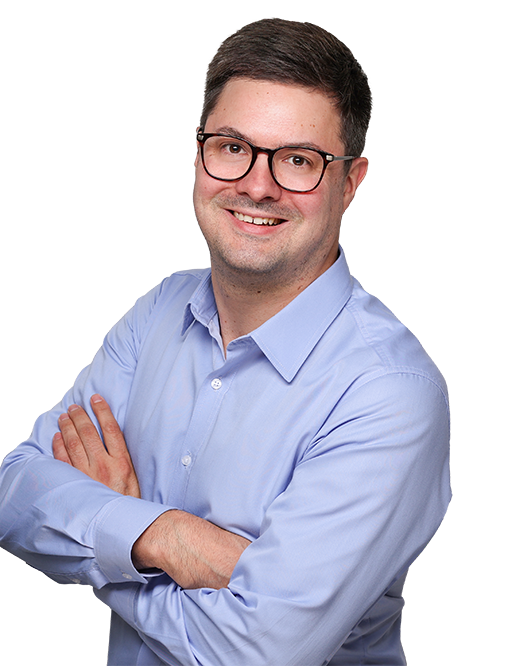
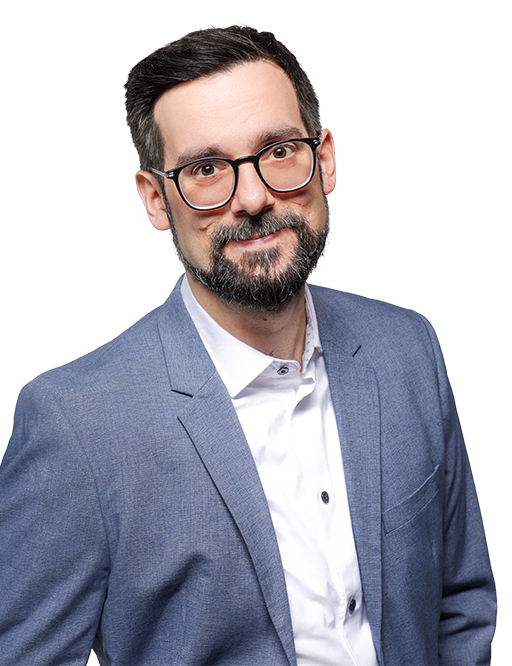
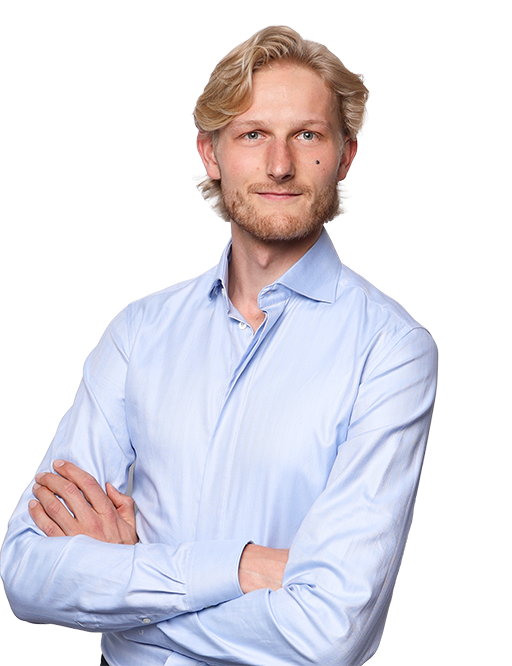
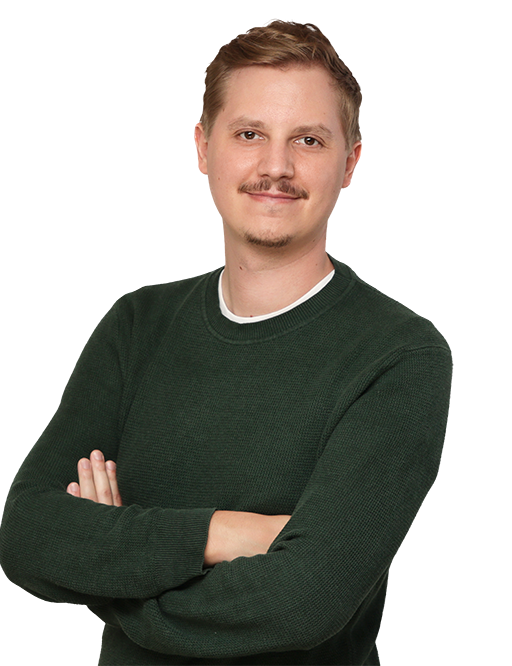
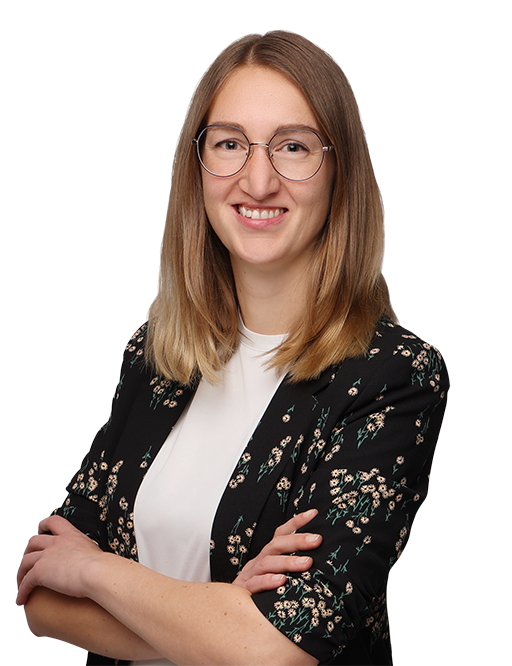
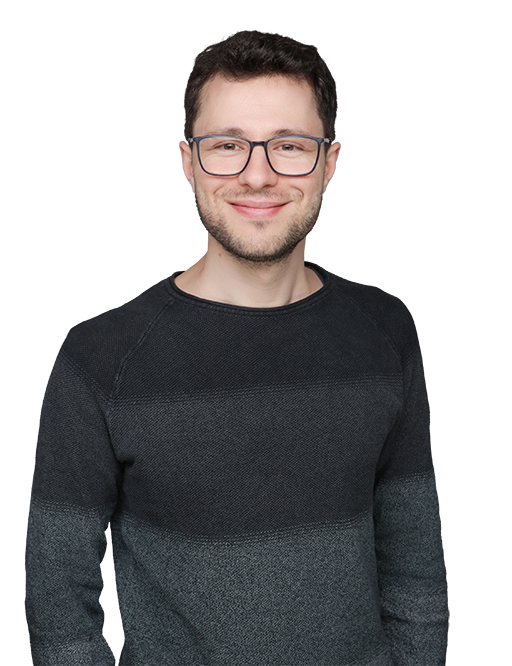
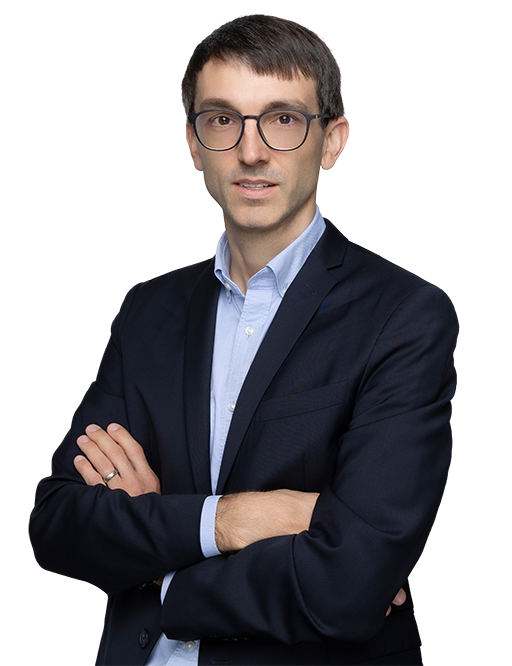
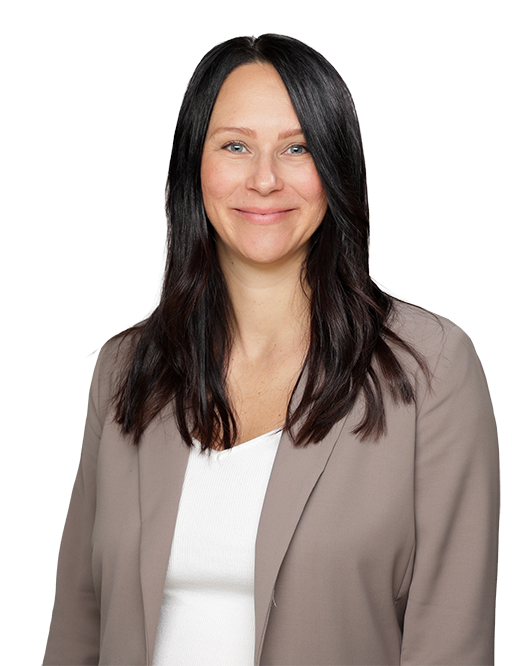
Find out more about our consulting and services:
-
Analyzing Digital Behavioral Data
Methods, tools, frameworks and infrastructures for analyzing digital behavioral data.
-
CSS Capacity Building
Talks, tutorials, materials on computational methods for the collection, processing, and analysis of digital behavioral data.
-
Digital Behavioral Data: Datasets
Curated digital behavioral data – datasets for scientific re-use.
-
GESIS Guides to Digital Behavioral Data
Expertise and hands-on advice on the acquisition and analysis of digital behavioral data and the computational methods needed.