The digitization of society brings about a structural change of the public sphere and the private, in which algorithms – quite literally – play a decisive role. We all interact in and with socio-technical systems, e.g. social media, search engines, online stores, job application platforms, news and information platforms. In these systems, algorithms play a major role in deciding which content, groups, people or institutions are presented or recommended to us and how they are being priorized. Algorithms often take the concrete behavior of users as a starting point and thus create a complex recursive interaction between the operating algorithm and human action or experience. With this, artificial intelligence, algorithms and automated processes create dynamics that might not be perceivable by users but do create social structures that substantially influence our individual lives and society as a whole. Whether or not these consequences are desirable can only be discussed and evaluated if we know precisely how digital technologies, the Web and the algorithms therein shape social structures.
This is why GESIS studies the mechanisms of socio-technical systems in order to understand the social change they bring about and to improve the basis for informed and "good" decisions. We do this through collecting digital behavioral data on societal issues, conducting online experiments to analyze behavioral patterns and their susceptibility in digital environments, and developing analytical tools. One of the most pressing social issues is inequality. Algorithms can reinforce existing social inequality or generate new distortions or discrimination. We investigate how distortions (e.g. gender bias) occur in digital practice and how, on the other hand, algorithms and AI can be used to counteract structural inequality and injustice or misinformation.
- Soldner, Felix, Bennett Kleinberg, and Shane Johnson. 2022. "Trends in online consumer fraud: A data science perspective." In A fresh look at fraud: Theoretical and applied perspectives, edited by Stacey Wood, and Yaniv Hanoch, 167-191. Binghamton, NY: Routledge. doi: https://doi.org/10.4324/9781003017189.
- Rosenbusch, Hannes, Felix Soldner, Anthony M. Evans, and Marcel Zeelenberg. 2021. "Supervised machine learning methods in psychology: A practical introduction with annotated R code." Social and Personality Psychology Compass 15 (2): e12579. doi: https://doi.org/10.1111/spc3.12579.
- Soldner, Felix, Justin Chun-ting Ho, Mykola Makhortykh, Isabelle Van der Vegt, Maximilian Mozes, and Bennett Kleinberg. 2019. "Uphill from here: Sentiment patterns in videos from left-and right-wingYouTube news channels." In Proceedings of the Third Workshop on Natural Language Processing and Computational Social Science, 84–93. 3rd Workshop on Natural Language Processing and Computational Social Science. doi: https://doi.org/10.18653/v1/W19-2110.
- Soldner, Felix, Veronica Pérez-Rosas, and Rada Mihalcea. 2019. "Box of Lies: Multimodal Deception Detection in Dialogues." In Proceedings of the 2019 Conference of the North American Chapter of the Association for Computational Linguistics: Human Language Technologies, Volume 1, 1768–1777. Association for Computational Linguistics (ACL). doi: https://doi.org/10.18653/v1/n19-1175.
- Sen, Indira, Fabian Flöck, Katrin Weller, Bernd Weiß, and Claudia Wagner. 2021. "A Total Error Framework for Digital Traces of Human Behavior on Online Platforms." Public Opinion Quarterly 85 (S1): 399–422. doi: https://doi.org/10.1093/poq/nfab018.
Title | Start | End | Funder |
---|---|---|---|
Political polarization and individualized online information environments: A longitudinal tracking study
(POLTRACK)
|
2022-01-01 | 2025-08-30 | SAW (Leibniz) |
NFDI for Data Science and Artificial Intelligence
(NFDI4DS)
|
2021-10-01 | 2026-09-30 | DFG |
Dehumanization Online: Measurement and Consequences (Professorinnenprogramm)
(DeHum)
|
2021-01-01 | 2026-09-30 | SAW (Leibniz) |
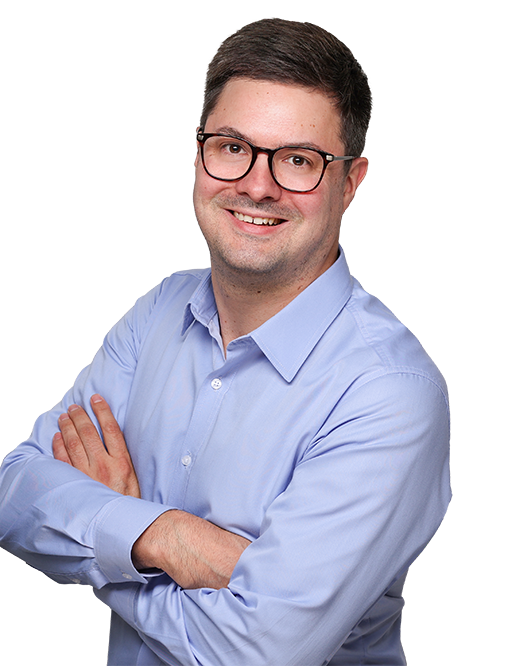
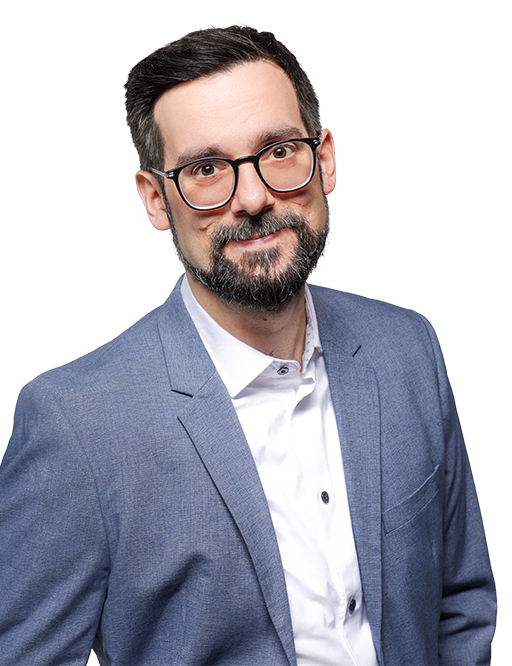
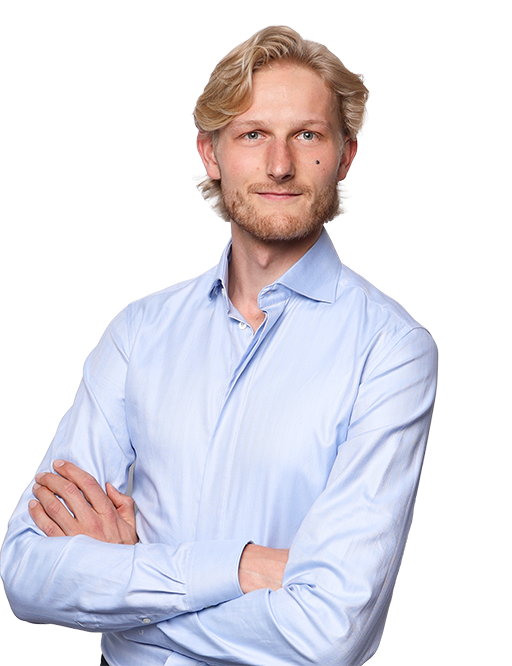
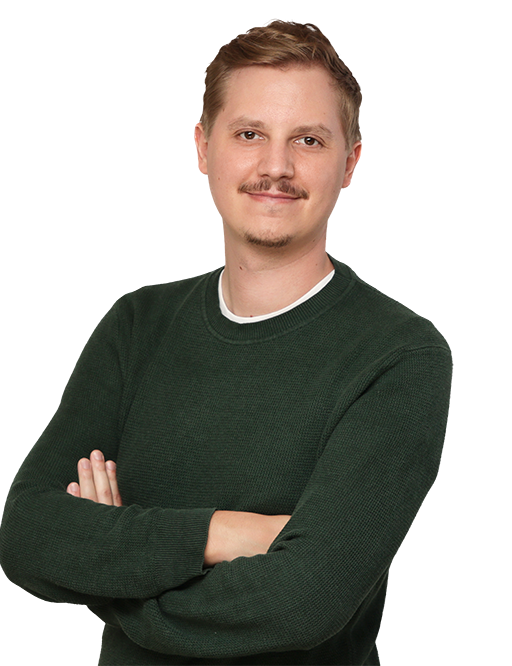
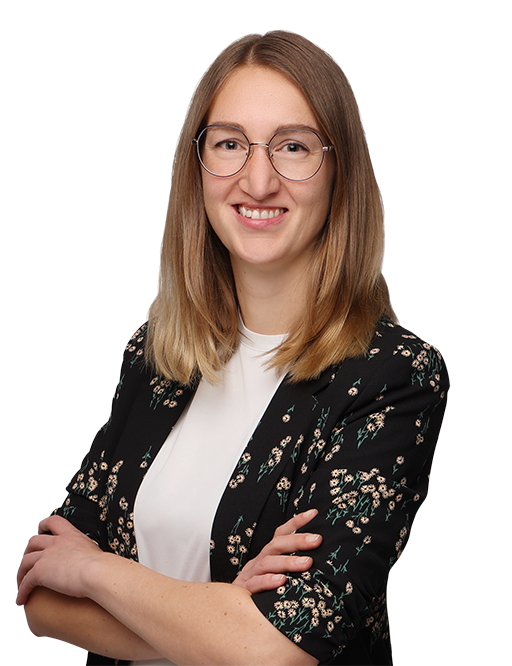
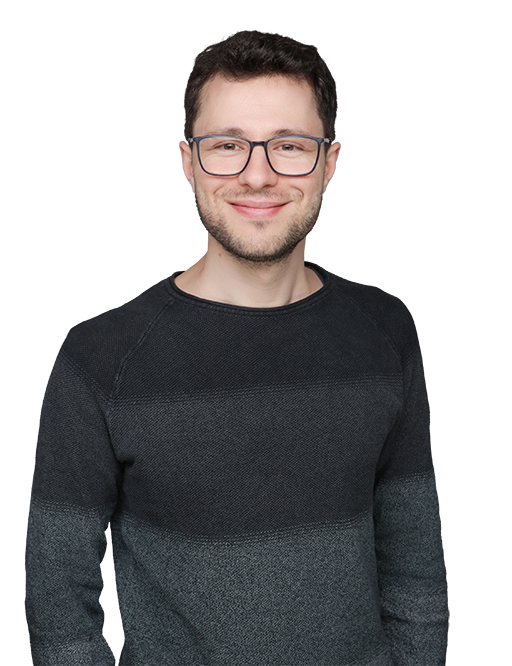
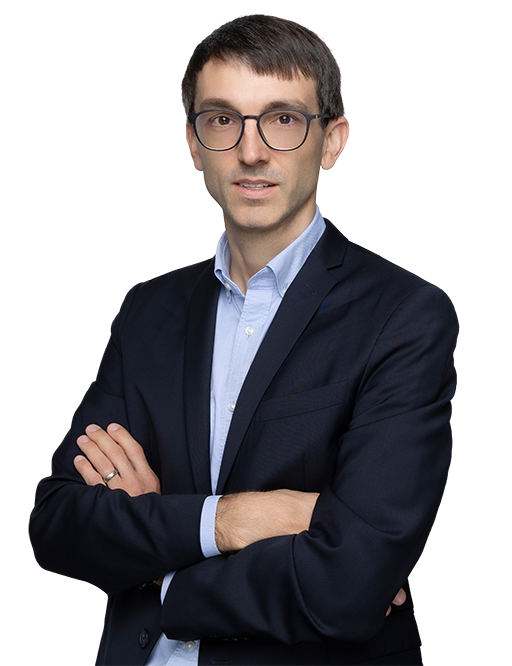
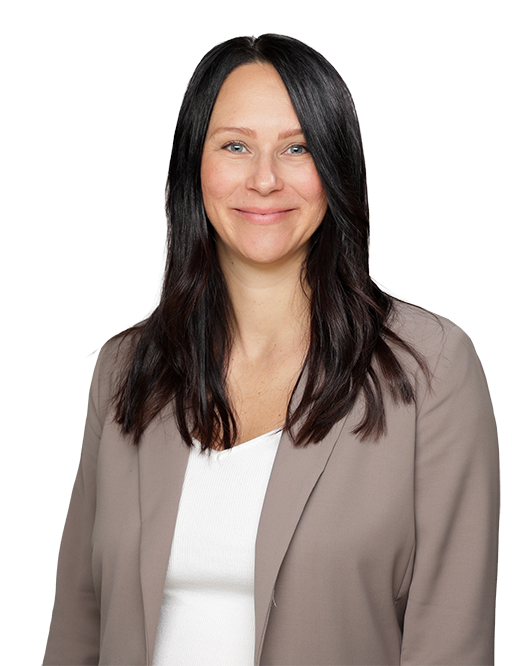
Find out more about our consulting and services:
-
Analyzing Digital Behavioral Data
Methods, tools, frameworks and infrastructures for analyzing digital behavioral data.
-
CSS Capacity Building
Talks, tutorials, materials on computational methods for the collection, processing, and analysis of digital behavioral data.
-
Digital Behavioral Data: Datasets
Curated digital behavioral data – datasets for scientific re-use.
-
GESIS Guides to Digital Behavioral Data
Expertise and hands-on advice on the acquisition and analysis of digital behavioral data and the computational methods needed.